As 5G, IoT (Refer article:4G-DTU, THE ESSENTIAL TOOL FOR IOT APPLICATIONS), and edge computing become more prevalent, the digital transformation of the network industry and enterprises becomes a reality, bringing new complexity to network operations - coexistence of old and new technologies, mixed networks, various frequency bands and spectrums, and a large number of connected devices. In addition, new requirements from IoT and industrial use cases require further performance enhancement and network optimization to reduce complexity, meet the requirements of new technologies and use cases, improve network performance, and achieve network automation.
navigation:
Five Advantages of AI in the Network
The Future of AI Network Applications
Five Advantages of AI in the Network
-
Efficiency
The real market benefit of AI is automating repetitive tasks, taking quick and effective action, and releasing employees or resources for other areas. By taking over daily tasks and improving network efficiency, AI-driven automation can optimize network operations and reduce costs. These algorithms can greatly improve capacity planning and optimization throughout the network. In operation, AI can more accurately identify the root cause of problems, shorten problem resolution time, and predict and prevent degradation or interruptions, reducing or avoiding costly downtime. They can even enable self-healing networks, reducing (and ultimately eliminating) the need for human intervention. -
Performance Improvement
As networks become more complex, maintaining high network performance that meets customer expectations may be a challenge. By leveraging AI, communication service providers (CSPs) can manage increasing complexity while improving the customer experience. By analyzing network traffic patterns and optimizing resource allocation, AI can improve throughput and reliability. By allocating dynamic networks and computing resources to regions with high service demand and optimizing data routing to reduce congestion, they can improve bandwidth and latency. -
Energy and Sustainability
With rising energy costs, the implementation of net-zero targets, and increasing capacity demands on networks, energy use has become critical to sustainable operations. While we may expect zero-energy devices in the future of 6G, AI can help address this issue by maximizing network utilization without compromising energy-saving functionality. AI can also autonomously act on real-time or even predicted traffic, helping CSPs reduce energy consumption and carbon footprints, contributing to sustainable development goals and lowering operating costs. -
Reliability and Security
Systems must have built-in interpretability and transparency, ensuring there is always enough information to explain, improve, or resolve issues, and ensuring that data entering the system is secure, not biased towards specific results that would lead to AI bias, and preventing any attacks. In AI, security and compliance are often areas of concern. But trustworthy AI algorithms can actually reduce security and compliance issues. By detecting and preventing security threats such as network attacks and fraud, AI can help CSPs protect their networks and customer data, and demonstrate their commitment to responsible business practices. AI algorithms can also be used for fraud detection and prevention in areas such as billing and user management, helping operators reduce losses and improve their financial performance. -
New Business Opportunities
By using AI in the network to gain insights from data and behavioral patterns, CSPs have a unique opportunity to gain deeper insights into their customers and their needs. It enables them to identify new business opportunities, provide targeted, personalized services to customers, and proactively take action based on their intent to meet consumer needs. If used effectively, these services can increase revenue and provide a competitive advantage in the market.
The Future of AI Network Applications
The decision regarding the shift of focus for AI network applications will be autonomously managed by the system through closed-loop self-interference, with cognitive technology having broad robustness and interpretability as a prerequisite for this autonomy. The development process of AI modules will also be automated through management pipelines and LCM. Moving along this technology trajectory, continuing to focus on the development, piloting, and deployment of the latest AI technologies in the AI field, will lay a strong, efficient, and intelligent foundation for emerging enterprise and consumer 5G revenue opportunities.
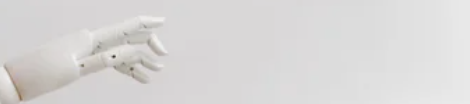